By Sarah Wunning and Satanjeev “Bano” Banerjee
Since the 1960s, GLIDE has relied on direct service volunteers to help provide safety net services (such as daily free meals) to the most vulnerable people in our community. Recently, we have been experimenting with a Skills-Based Volunteering (SBV) model, in which we use the specialized skills of volunteers to build capacity in different parts of the organization. At the same time, over the last decade, there has been an increase in San Francisco–based technology companies looking to engage with the local community, with employees eager to volunteer their skills to make an impact.
Twitter is one such company. Located only a few blocks from GLIDE, the company organizes a volunteer day for all of its staff twice a year called Twitter For Good Day (TFG). Traditionally, most TFG Day volunteering projects have been direct service in nature, such as serving meals at GLIDE and other similar nonprofits, cleaning parks, or assembling hygiene kits. In addition to these worthy projects, Twitter and GLIDE’s Data, Strategy and Evaluation team have partnered over the last couple of years to develop data projects that utilize volunteers with data skills.
The Challenges and Our Approach
Using the typical short-term volunteer model for data analysis comes with some unique challenges. Unlike many direct service volunteering projects, data volunteers need more context about the task, the data, and what kind of analysis will most benefit the organization.
Enabling short-term volunteers to do data analysis within three hours means doing a lot of prep work beforehand. Unfortunately, just as GLIDE staff often does not have adequate time for the actual analysis, there is also a shortage of bandwidth for the prep work. Our approach to tackling this challenge is to rely on one or two long-term volunteers to help with the data prep, including time-consuming tasks like data cleaning, anonymizing, setting up the logistics for the day, and recruiting volunteers from Twitter.
Coming up with the right type of project or question is also an important part of preparing for skills-based volunteers. The right balance of a project—one that is important but not urgent, impactful for volunteers to work on, and can be accomplished in three hours—can be difficult to find. Over the years, we have found that asking open-ended questions and mixing in questions that are not purely related to data analysis has created the most value for both GLIDE and the volunteers working on the projects.
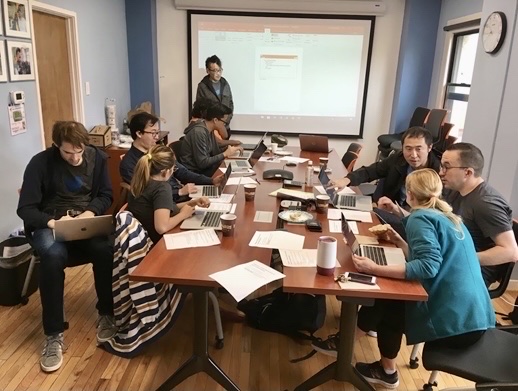
“Data for Good” — some highlights
Over the last three years, dozens of skills-based volunteers have worked on various projects at GLIDE. We call these joint efforts Data for Good. Below, we showcase just two of our several collaborative projects:
Analyzing demographics and who exactly GLIDE serves
GLIDE program staff have noticed GLIDE’s participant population demographics change over the years, and they wanted to know what the data shows—in particular, if there are certain population groups within the Tenderloin that are underserved by GLIDE’s programs. Volunteers received anonymized GLIDE program data, which they then sliced and diced by demographic information, such as age, gender, race, sexual orientation, etc. They then compared that information to similar San Francisco below-poverty census data and Tenderloin census data, and found that, overall, there were not any major gaps between GLIDE’s program participant data and the census data, and that trends were consistent between GLIDE and the Tenderloin neighborhood. This useful information has inspired GLIDE to redo this analysis on an ongoing basis, in order to identify any potential future gaps in service to the community.
Getting at an Unduplicated Client Count for the Daily Free Meals Program
GLIDE serves three meals a day, 364 days a year. In order to remove barriers to food security, participants don’t sign in or fill out forms and clients can receive as many meals as they want in a day or mealtime. The only data we do have is the total number of plates served. A question that GLIDE has always been interested in answering accurately is: How many unique participants come to GLIDE’s Daily Free Meals in a year? We presented volunteers with this open-ended question to have them brainstorm possible solutions. GLIDE staff showed volunteers how a meal shift is run and how participants flow through the dining hall. The volunteers proposed using a lightweight survey coupled with statistical modeling to arrive at an accurate answer to this question. We are now following up by piloting these lightweight surveys, conducted while participants wait in line.
Twitter folks volunteering their data science skills to analyze data at @GLIDEsf for #TwitterForGood #DataScienceForGood! pic.twitter.com/NgTX5NFtrU
— Satanjeev Banerjee (@satanjeev) May 18, 2018
What We Learned
Data for Good has had six iterations so far. Across these sessions, we have learned various lessons that have helped us improve the program. We list them below in the hope that these learnings will be useful to other nonprofits.
- Champions Needed: Skills-Based Volunteering isn’t a common volunteering model for either nonprofits or for-profits, partly because it is difficult to do well. In order for SBV to be successful, it’s necessary to have people on both sides that are committed to its success over a long period of time.
- Patience: With direct service volunteering, you often see immediate impact, whereas with SBV, it takes time to build up impact. For example, in the demographics analysis project described above, the first round of Data for Good activity answered some questions and laid the groundwork for future analysis. By doing more follow up analyses, we have built on this work over the course of several years and deepened our understanding of GLIDE’s program participants.
- Think Beyond Data: In the beginning, we thought narrowly about the types of projects that are suitable for Data for Good—mostly pure data analysis. Over the years, however, we have expanded to other kinds of SBV, such as creating slide decks from data reports, performing simple data clean ups, and conducting internet research. These kinds of projects are as impactful as data analysis, and volunteers enjoy getting to use skills outside of data analysis.
Skills-based volunteering or SBV has been an impactful addition to GLIDE’s volunteering program. It has allowed GLIDE to not only answer some longstanding questions but has also deepened our relationship with Twitter. Through this relationship, we have started working on other long-term projects, such as running our first in-house randomized controlled trial on the number of mail solicitations sent to donors. In the future, we hope to expand this model to other departments across GLIDE—and we hope that other nonprofit organizations and for-profit companies will also join us on this journey!
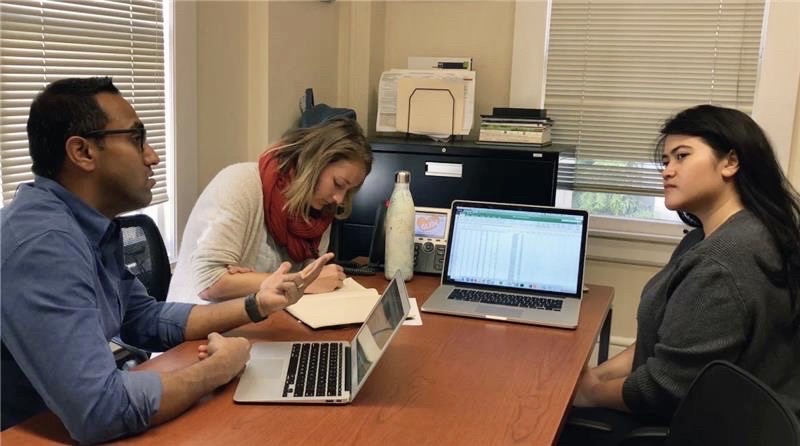
Sarah Wunning (center) is GLIDE’s Data Systems Manager and Satanjeev “Bano” Banerjee (left) is Machine Learning Engineer at Twitter. They would like to thank the following colleagues for their generous support of the work detailed above. At GLIDE: Kate Purdy and Caitlin Jolicoeur. At Twitter: Kania Azrina, JP Wong, Bhargav Manjipudi, London Lee, and Karl Robillard.
And, not least, thank you to all the Twitter volunteers who’ve participated in Data for Good over the last several years, we appreciate you!